Principle 6: Profiling
Avoid Unfair Use of Profiling
Many companies and states create very detailed and precise profiles of you, for many different reasons. Some uses of profiling are completely unproblematic, while others are very unfair. The Principle of Profiling is simple: Avoid Unfair Use of Profiling.
What is Profiling?
Profiling often refers to the automatic processing of data about a person, often with the intention of making statistical predictions about the person. In order to make precise profiles, companies and states collect huge amounts of data. Statistical algorithms (data analytics) are employed on the data in order to find patterns.
These patterns can be used to predict whether people are likely to buy a certain product, vote for a certain political party, commit a crime, and so on. In short, profiling is used to make all sorts of important decisions which can have a very significant impact on people’s lives.
When is Profiling Problematic?
Profiling is not problematic in itself. We all make profiles of eachother in our minds all the time. We get information about each other, and we automatically form opinions and make predictions about each other all the time. So when is profiling problematic? Simply put, profiling is problematic when it is used in a harmful or unfair way. Let us look at an example.
An Example
Imagine that Smith and Peter both apply for long-term loans in the same bank, in order to buy new homes for their respective families. They both have the same age, the same income, and so on. In order to decide whether they are eligible for the loan, the bank profiles Smith and Peter. The algorithm recommends that Smith is not eligible for the loan, while Peter is.
Smith complains to the bank advisor Jones, explaining that he and Peter have the same age, the same income etc., so he doesn’t understand why he is not eligible why Peter is. Jones explains that historically, people living in Smith’s neighbourhood tend to have a bad track record when it comes to paying back long term loans. This, and only this, is the reason why the algorithm declined Smith’s application but approved Peter’s.
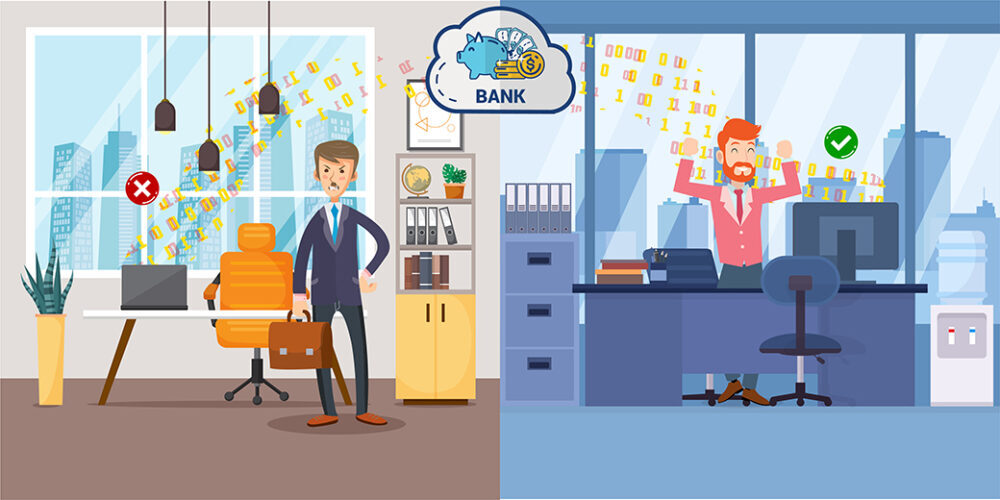
Fairness
Most people will probably agree that the way profiling is used in the example above is unfair. The profiling of Smith and Peter is not problematic in itself, but it is used in a discriminating and unfair way, by treating them differently due to how people in Smith’s neighbourhood have behaved in the past.
All things being equal, profiling should be used fairly in a way that treats like cases alike. If profiling is used to determine whether someone is eligible for a loan, it should treat people of similar age and similar financial circumstances alike. Similarly, if profiling is used to determine whether someone is eligible for a life-saving medical procedure, it should treat people with similar symptoms and health conditions alike, and so on.
When humans make decisions, they are not always treated like cases alike either. Humans are biased in all sorts of ways. Profiling can help decrease biases, but profiling can also confirm or amplify biases.
Biased Algorithms
Besides treating like cases alike, the algorithms used for profiling should not confirm or amplify biases. In the example above, it might be true that people in Smith’s neighbourhood tend to have bad track records when it comes to paying back long term loans. But the neighbourhood itself is probably not the real cause of it. It’s more likely that some people with bad track records tend to move to that neighbourhood for some other reason, for example, that it’s cheap. When Smith’s application is turned down, the algorithm confirms that people from that neighbourhood should be denied loans.
As much as possible, profiling algorithms should be based on real causes, rather than on spurious correlations with factors like location, race, ethnicity, physical appearance etc. In general, we must avoid the unfair use of profiling.
Avoid unfair use of profiling.